- Algorithmic Trading
- Unlock the potential of algorithmic trading with advanced strategies, backtesting, and data analytics. Learn how KX's cutting-edge platform empowers traders to optimize performance, reduce risks, and capitalize on real-time market opportunities. Read More
- Backtesting
- Optimize trading strategies with backtesting: analyze past performance, refine approaches, and achieve future success. Read More
- Counterparty Risk
- Learn everything about counterparty risk, its impact on financial institutions, and how to backtest effective models. Discover key risk types, mitigation strategies, and advanced CCR solutions with KX. Enhance your risk assessments and safeguard profitability. Read More
- Credit Risk Analysis Modeling
- Credit risk modeling allows financial institutions to better manage their portfolios by measuring the risk of loss. Learn more by reading our article. Read More
- Data Architecture
- A strong data architecture is crucial for effective and efficient retrieval and analysis of enterprise big data. Read More
- Data Structures and Algorithms
- Learn the fundamentals of data structures and algorithms, key concepts, and how they optimize performance in software development. Read More
- Forex (Foreign Exchange) Analysis
- Analyze and predict relative movements in currency prices to better optimize your forex portfolio. Learn more by reading our guide to forex analysis. Read More
- FX Trading Analytics
- Discover how FX Trading Analytics empowers enterprises with AI, real-time insights, and data-driven strategies to succeed in volatile forex markets. Read More
- High-Dimensional Data
- How high-dimensional data can be effectively managed with real-time analytics. Read More
- Hyperparameters
- Explore hyperparameters, tuning techniques, and optimization methods to enhance machine learning model performance. Read More
- LLM Agents
- Discover how specialized agents enhance LLMs, enabling advanced functionality, tailored responses, and transformative applications. Read More
- LLM Architecture
- LLMs have revolutionized the world. Curious about how they function? Discover the architecture of large language models. Read More
- Momentum Ignition
- Learn about momentum ignition, its role in trading strategies, and how enterprises leverage AI to stay ahead. Read More
- Multimodal Retrieval Augmented Generation (RAG)
- Imagine AI combining text, images, and data seamlessly. Unlock the power of Multimodal RAG in this quick guide. Read More
- P&L Attribution Analysis
- Master P&L attribution analysis to enhance risk management and financial performance. Learn how KX's AI-ready analytics platform streamlines P&L analysis, backtesting, and credit risk model validation for actionable insights. Read More
- Quantitative Trading
- Quantitative trading involves the use of complex mathematical models to guide investment in financial markets. Read More
- Semantic Layer
- How the semantic layer enhances real-time analytics and transforms business intelligence. Read More
- Similarity Search
- How similarity search uses AI and real-time analytics to identify visually similar images across industries like finance, manufacturing, and more. Read More
- Streaming Analytics
- Explore how streaming analytics delivers real-time data insights for faster decisions and enhanced enterprise performance. Read More
- Structured vs. Unstructured Data
- Explore the differences between structured and unstructured data, their uses, and how they impact data storage and analysis strategies. Read More
- Time Series Database
- Discover the power of time series databases (TSDBs) for managing and analyzing time-stamped data efficiently. Read More
- Time series foundation models (TSFM)
- Learn how time series foundation models (TSFMs) are rapidly changing how we handle and predict time-stamped data. Read More
- Trade and Market Surveillance
- Trade surveillance involves monitoring financial trades to ensure compliance with the internal and external regulatory framework. Read More
- Trading Analytics
- Real-time trading analytics involves performance analysis based on today's and historical trading data, allowing for faster, more profitable decisions. Read More
- Transaction Cost Analysis
- How TCA helps you analyze trade prices and validate whether you’re trading with the greatest efficiency. Read More
- Unified Analytics
- Learn what Unified Analytics is, how it works, and why it’s essential for modern enterprises seeking smarter, data-driven decisions. Read More
- Vector Database
- Discover what a vector database is, how it works, and its key benefits for handling complex, high-dimensional data efficiently. Read More
- Vector Embeddings
- How vector embeddings transform data for AI and machine learning. Read More
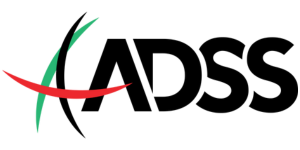
Capital Markets
Read More
About ADSS
ADSS leverages KX real-time data platform to accelerate its transformational growth strategy.