by Steve Wilcockson
When the artist most synonymous with Ragtime, Scott Joplin, learnt the piano, serious musical circles felt Ragtime was too “Tin Pan Alley.” Joplin would blend styles and formalize compositions that would be re-played with the same fidelity as when he wrote them, for example, The Entertainer.
So, what do Scott Joplin and Ragtime have to do with Generative AI tech and KDB.AI Server Edition?
First, Generative AI must be carefully teased, like the notes of Scott Joplin’s The Entertainer. “Playing the right notes in the wrong order,” is akin to a GenAI-inspired hallucination that could lead to an adverse outcome: a flash crash, or a mistimed medical or political intervention.
Second, the “Ragtime” genre of yesteryear plays nicely with the RAG Time advantage of KDB.AI Server. RAG represents the Retrieval Augmented Generation orchestrated Generative AI workflow, while KDB.AI provides a natural time-based advantage.
A Vector Database Built for RAG and Time
RAG is tech’s favored approach for incorporating enterprise data into Generative AI workflows. Enterprise data stores of vector embeddings, brought to life through fast semantic search in KDB.AI, accompany Foundation and Large Language Models orchestrated through development tools like LangChain.
Organizations that implement RAG can deploy data-sets and provide guardrails around prompts and responses mitigating against LLM “hallucinations.” Like how Scott Joplin intended his compositions to be played – not over-improvised – enterprises require their AI infrastructures, particularly LLM prompt responses, to provide similar fidelity.
Below, “Figure 1” shows KDB.AI in a simple Naïve RAG scenario. RAG has two primary steps:
- “Retrieval” where, based on the query, additional related information is extracted from internal sources
- “Generation” where that additional data is passed and augmented along with the original question to the LLM for generating a response
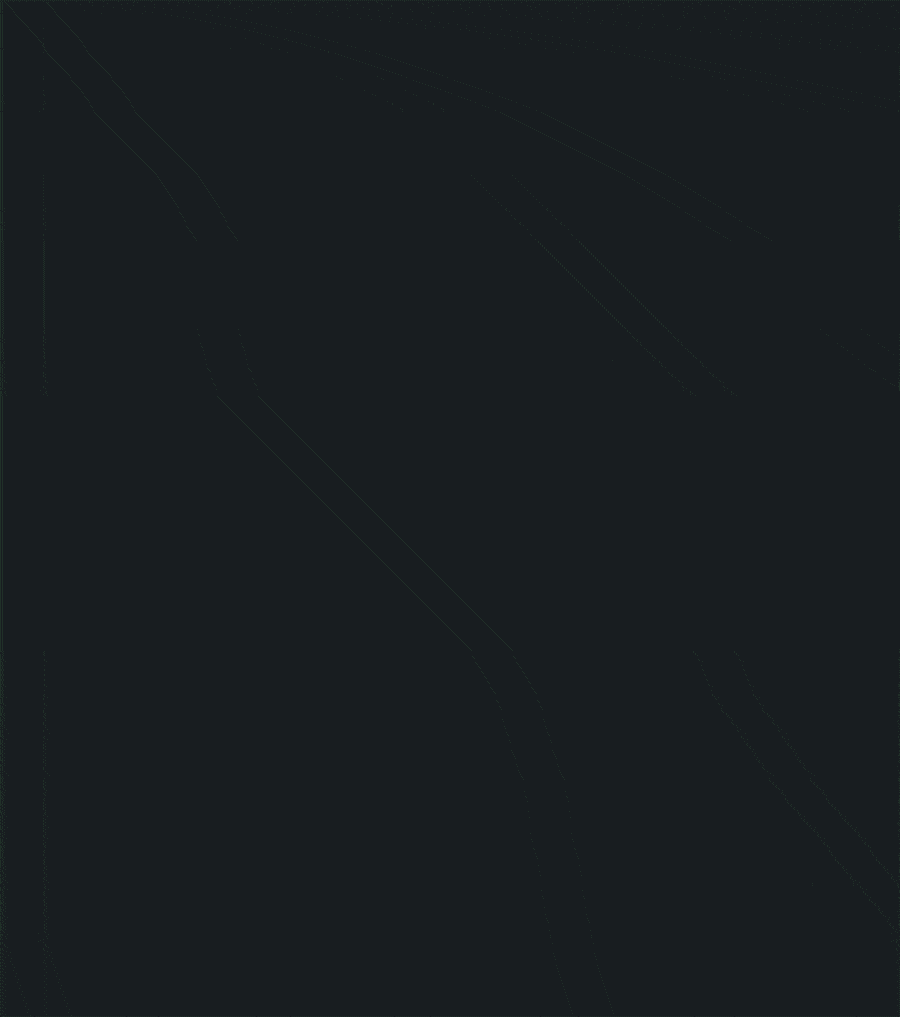
Fig 1. Simple naïve RAG architecture
With RAG, the vector embeddings saved in the KDB.AI vector database, digital encodings of text, videos, pdfs, or other data, provide information your LLM didn’t have access to when it was trained initially. For example:
- Private, internal information such as contacts, internal policies or personnel records
- Corrections or updates to previously published information or official records
- Price changes, news updates or corporate action events that have occurred in the interim
With this vector knowledge store, you can explore semantic search in conjunction with your LLM environment, orchestrating with LangChain or your own data pipeline tool.
What about time? KDB.AI is built for time, incorporating temporal information into prompt responses through filtering, time windowing, and search over time.
Pre-trained models like GPT, which stands for Generative Pre-trained Transformer, are static models tied to the time at which they were trained. Handling and responding to data and analytics over time, including real-time, underpins the core technology on which KDB.AI is based. This makes the KDB.AI vector database, working alongside the pre-trained static foundation models, well suited to temporal use cases at all levels of granularity.
Why KDB.AI Server?
KX recently released KDB.AI Server, a highly-performant, scalable, vector database for real-time contextual AI that runs on-premises, hybrid, or in the cloud with a single container deployment. In short, KDB.AI Server takes the freely available developer capabilities from KDB.AI Cloud Edition, and helps CDAOs, CTOs, and their business-facing teams build fast and run their data anywhere, in secure, enterprise, or customer-managed deployments. Vector database functionality is ported to the enterprise architecture of choice. You can:
- Create an index of vectors (Flat, IVF, IVFPQ, or HNSW)
- Append vectors to an Index
- Perform fast vector similarity search with optional metadata filtering. Available distance metrics include:
- dotProduct (similarity or alignment between two vectors)
- L2/Euclidian (measures straight-line distance between two points in Euclidean space)
- Cosine (assesses similarity in terms of the direction of the vectors)
- Persist an index to disk
- Load an index from disk
- Integrate with foundation and large language models through developer tooling such as LangChain and LlamaIndex, and with the KDB.AI ChatGPT Retrieval Plug-in
Note the deployment benefits of KDB.AI Server. KDB.AI’s efficiency in filtering and searching vector datasets, deploying unlimited numbers of time windows, and no performance hits, means less need for GPUs and other expensive hardware. You can deploy to your existing hardware, on-premises and/or on cloud, in conjunction with your enterprise data resources and production environments.
RAG Time Examples
Let’s explore some time-related prompts to understand the use cases KDB.AI can be applied to. Fig 2 shows a prompt response asking, “What products did Apple release in 2020?”
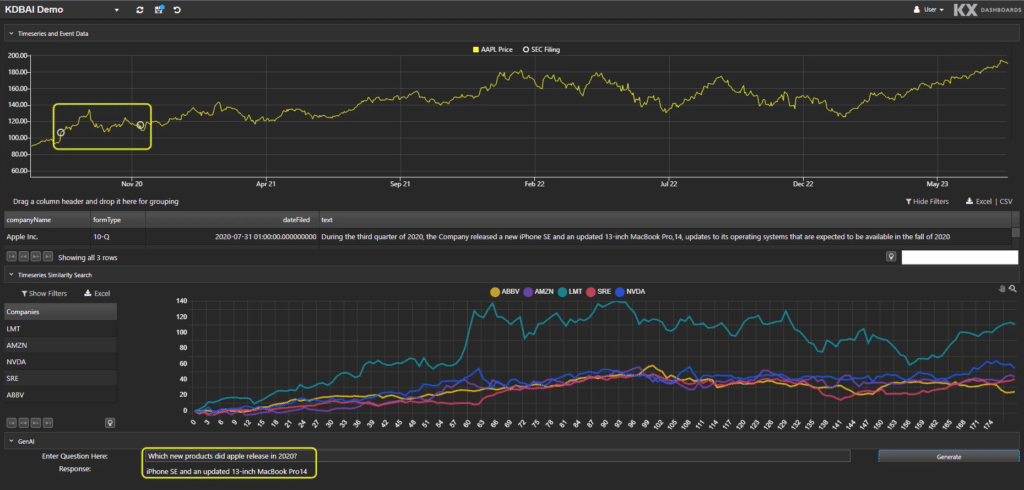
Fig 2. What products did Apple release in 2020?
The answer is returned in the bottom left: “iphone SE and an updated 13 inch Macbook Pro14.” If we’re focussed on market activities, we can now ask “What was the response of the share price during the period?”
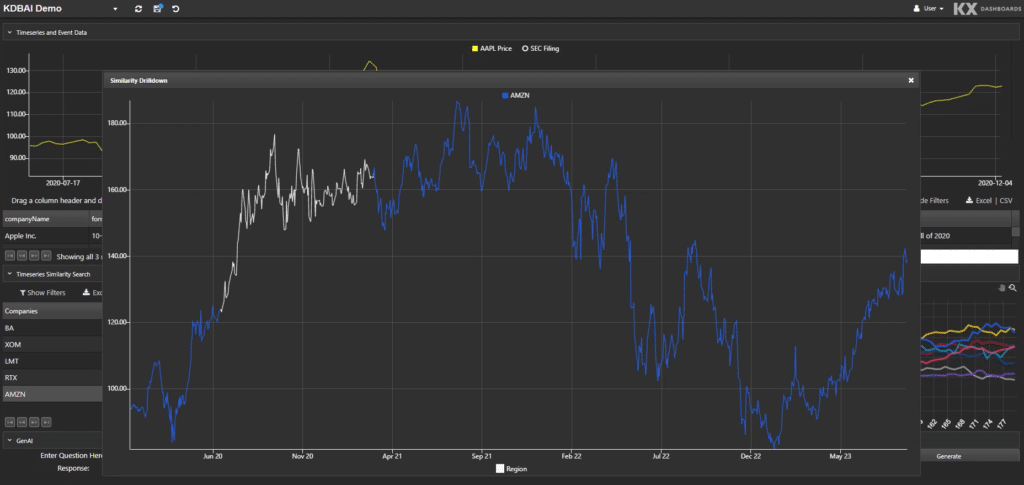
Fig 3. What was the impact to Apple’s share price?
Our queries can extend further. KDB.AI can recognize patterns and provide charts, highlight key source release information from original documents, quantify impacts, and compare with similar trends from prior years or different releases.
Were we a retailer with similar interests in Apple launch metrics, holding our own and industry-aggregated retail data-sets, instead of assessing the impact on share price, we could probe the impact on sales. We may, for example, compare our own sales figures, including the most recent ones, alongside the aggregate market knowledge “known” by the LLM. We might then benchmark our company’s sales to others, identify and compare to similar launches back in time, or to launches from Apple’s rivals.
Let’s now consider a more elaborate financial workflow example. Consider an investment strategy that trades BitCoin based on streaming sentiment, for example around regulation. A vector database with an LLM in a RAG architecture provides an excellent alternative to off-the-shelf black-box sentiment feed providers, of whom there are many. We can join social media feeds with price data and assess how regulatory announcements impact price, e.g. BTC.
Step 1:
- Identify news about a specific corporate entity and a government regulator from the news feed
- Use the LLM with an embeddings strategy to create vector embeddings stored in KDB.AI Server Edition
- Find time windows where a specific corporate entity (BTC, Bitcoin, bitcoin.org) is mentioned in the context of a regulator (SEC, FINRA, Fed, etc.)
Step 2:
- Determine if sentiment is positive or negative using natural language syntax
- Identify price movements in KDB.AI tick history during the time windows from step 1
- Use the selected LLM, e.g., Anthropic Claude or GPT and a RAG architecture to identify sentiment using vector embeddings with KDB.AI
A sample RAG architecture is shown in Figure 4, below.
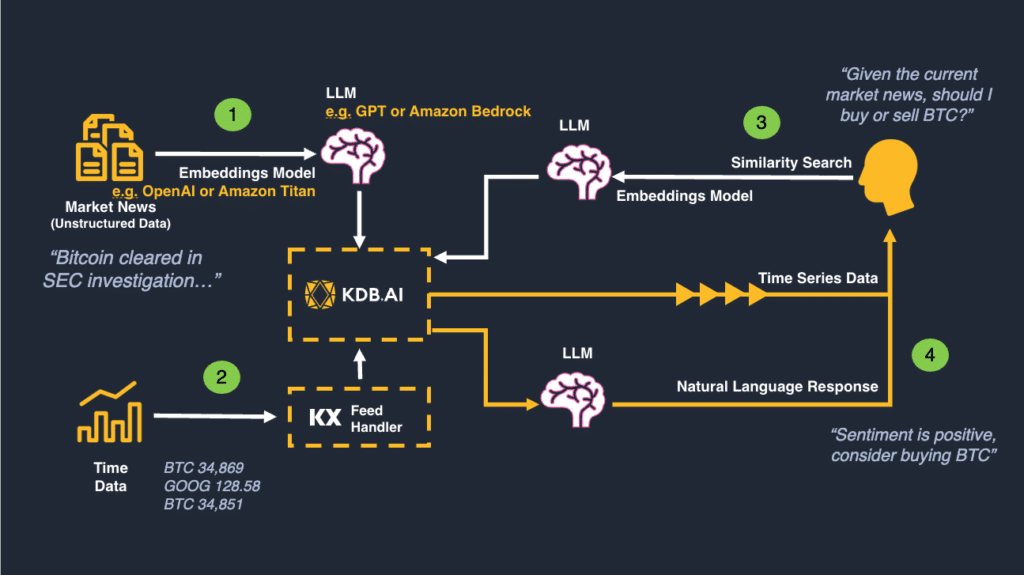
Figure 4: A Market-Driven Investment Model Process: Regulation Sentiment & BTC
With KDB.AI and a RAG architecture, we can quickly build and deploy a sentiment and price infrastructure, leverage information known by the LLM, guardrail, and make valuable with our own price and trade data to inform the investment strategy.
Banishing the RAG Time Blues
With your chosen LLM and RAG architecture, KDB.AI can leverage your knowledge base and provide insightful responses with speed, efficiency, and security, leveraging time to facilitate decision-making. And like the Ragtime of old, guardrail and provide structure to ensure your AI works as intended, like how Scott Joplin wanted pianists to reproduce his pieces with fidelity.
When running time-based queries, KDB.AI Server helps deploy AI routines with enterprise data-sets within your own secure firewalls – like our exemplified proprietary trading or retail data-sets alongside stores of unstructured data, e.g. analyst reports.
In our two earlier examples, we highlighted windowing and temporal analysis. Whatever your industry, be assured there is a time-based use case for you, for example:
- Telecommunications: Optimize capacity and coverage, deliver control at the edge, and maintain critical services with self-organizing networks
- Manufacturing: Quickly spot real-time predictive maintenance anomalies for smarter and more efficient plants
- Energy and Utilities: Analyze billions of data points instantly for grid and resource optimization and improved customer experience
- Aerospace and Defense: Analysis of operational data for correlation of intelligence, improving command decision making
- Healthcare: Model disease and interventions in virtual clinical trials to predict patient responses and required treatment dosages
Alternatively, please visit our dedicated Learning Hub to learn more about vector databases.