Key Takeaways
- Speed alone isn’t enough — hedge funds need high-fidelity, timely insights to make smarter, more informed trading strategies.
- Fast data prioritizes raw speed, while timely data ensures accuracy, context, and relevance for strategic decision-making.
- Integrating real-time and historical data allows hedge funds to generate richer, more predictive insights for market positioning.
- Predictive models thrive on high-fidelity, time-stamped data, improving risk detection and trading strategy precision.
- A seamless, integrated data ecosystem helps hedge funds align speed, accuracy, and timeliness to maximize alpha generation.
In financial markets, speed is often heralded as the ultimate competitive advantage. Many market-making firms, particularly those engaged in high-frequency trading (HFT), invest heavily in ultra-low latency systems designed to process vast volumes of data in milliseconds—aiming to react to market signals faster than their competitors. But for hedge funds, high-performance analytics isn’t just about being the fastest; it’s about being the smartest.
The real differentiator? High-fidelity, timely insights—data that is not only fast but also accurate, context-rich, and actionable when it matters most.
While speed enables rapid reactions, reaction without precision is just noise. The most successful hedge funds recognize that strategic, well-timed decisions — rooted in deep, contextual intelligence — consistently create more sustainable alpha than raw speed alone.
Understanding the difference between fast and timely data
While speed in data processing has obvious benefits, it is crucial to distinguish between fast data and timely data:
- Fast data: Prioritizes speed above all, often delivering large volumes of raw information with minimal filtering or context
- Timely data: Provides the most relevant, context-aware insights when they are needed for decision-making
For hedge funds, this distinction is critical. Acting on fast but incomplete data can lead to misaligned trades, exposure to adverse selection, and inefficient market positioning. Timely data—aligned with historical trends, liquidity conditions, and real-time market signals—enables smarter execution strategies that optimize spreads and mitigate risk.
Firms that integrate real-time analytics with pre-trade decision support, post-trade analysis, quant research, and historical pattern detection can better navigate shifting market conditions, improving execution quality and strategic alignment.
The role of time series data in hedge fund analytics
Timely, high-fidelity insights come from granular, accurate, and contextually relevant data. In hedge fund analytics, this means:
- Granular data capture: Tick-level insights provide a clearer picture of order flow, spread movements, and market liquidity, allowing for precise modeling and forecasting
- Contextual accuracy: Point-in-time accuracy eliminates look-ahead bias during backtesting and ensures that live trading strategies reflect real-world conditions
- Consistency across data streams: Integrating disparate datasets into a cohesive framework enables comprehensive analytics that inform robust trading strategies
Market makers thrive by capturing fleeting opportunities. Time series analytics enable firms to process both historical and real-time data simultaneously, detecting market shifts, optimizing order execution, and gaining a competitive edge.
Without this, even the fastest trading systems fall short — decisions made on incomplete or inaccurate data lead to suboptimal outcomes.
How hedge funds can align speed, fidelity, and timeliness
The most successful approach is to balance speed, fidelity, and timeliness to generate smarter insights. High-performance time series analytics bridge the gap between historical context and real-time decision-making. Here’s how:
1. Strengthen pre-trade analytics with real-time and historical data
Combining real-time market feeds with extensive historical datasets allows firms to generate context-rich insights. By aligning historical patterns with live data, hedge funds can identify opportunities and risks in real time, enhancing decision-making and execution.
This approach supports:
- Pre-trade signal validation, reducing false positives in trade execution
- Market impact forecasting, helping traders time execution optimally
- Volatility-adjusted trading models, integrating historical variance into real-time decision-making
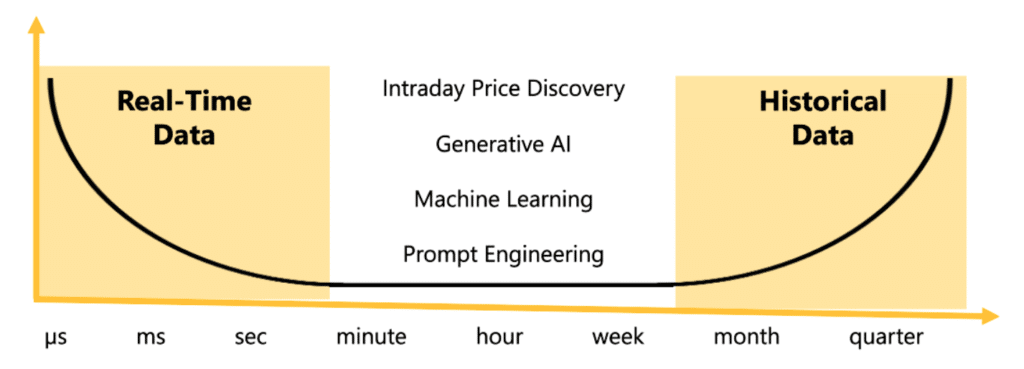
2. Optimize data pipelines for dynamic processing
Flexible data architectures enable dynamic scaling based on market conditions. Real-time streaming analytics ensure that the most relevant data is processed first, rather than overwhelming teams with unfiltered market noise.
This capability is essential for:
- Backtesting and model validation, ensuring strategies remain adaptive
- Post-trade analytics, evaluating slippage, execution costs, and liquidity conditions
- Regulatory and compliance reporting, maintaining auditable trade histories
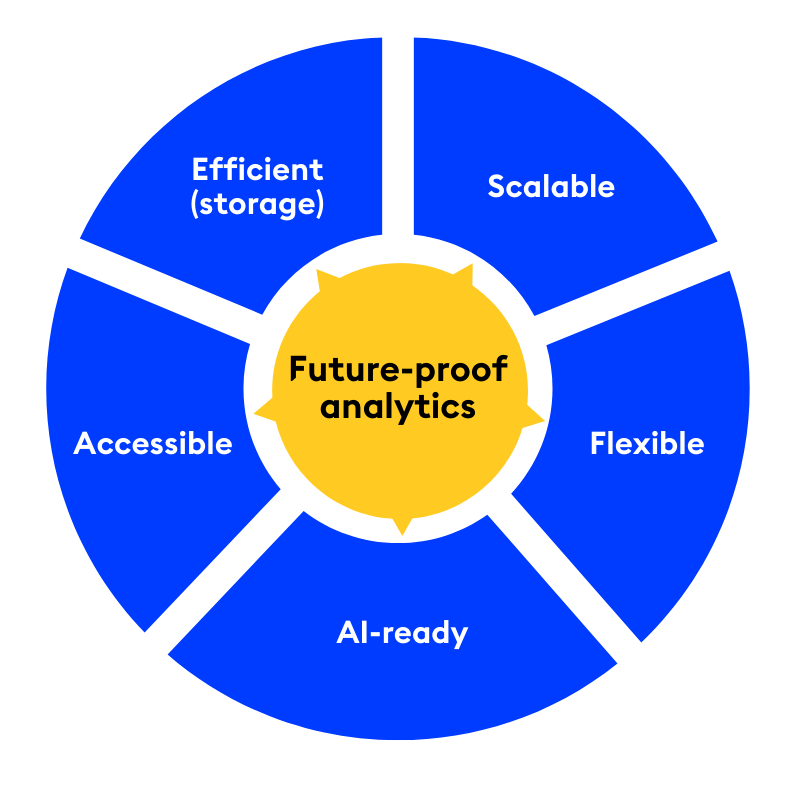
3. Enhance predictive analytics with pattern and trend detection
Machine learning models thrive on high-fidelity time series data. With well-structured datasets, you can detect patterns and generate reliable forecasts—moving from reactive to proactive trading strategies.
Comparing different time periods using accurate, time-stamped data helps firms:
- Identify emerging trade signals before they become widely recognized
- Enhance portfolio hedging strategies by detecting shifts in asset correlations
- Improve trade allocation models, using historical patterns to inform future execution
AI doesn’t predict markets with certainty, but it augments decision-making by identifying patterns that traditional models might miss.
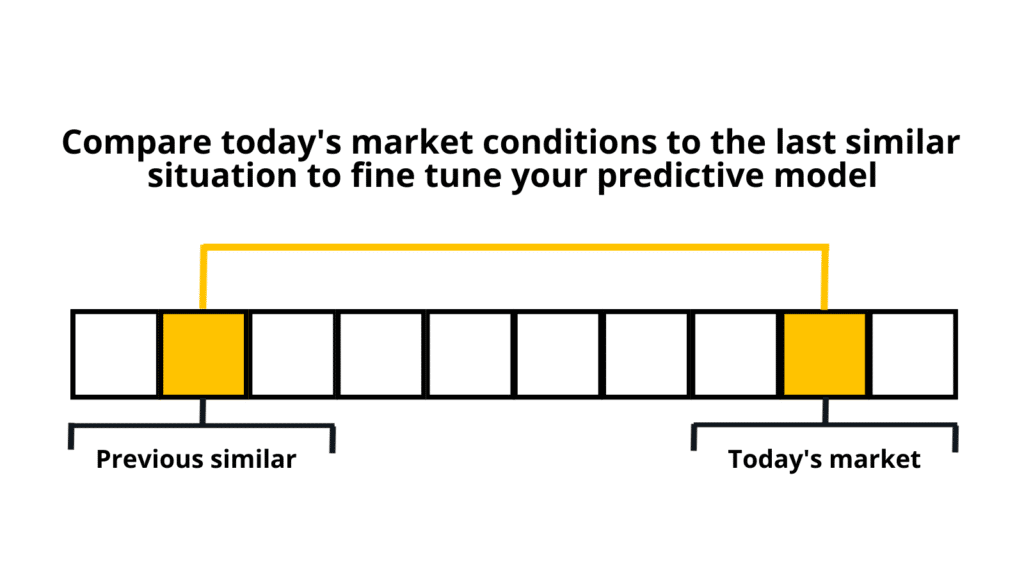
4. Ensure seamless data integration across teams
A unified data ecosystem eliminates silos, ensuring that all teams — traders, quants, and risk managers — access consistent, real-time insights. This consistency reduces the chances of errors and accelerates the decision-making process across your organization.
This is particularly valuable in:
- Quant research, where model assumptions depend on high-integrity datasets
- Risk analytics, ensuring exposure calculations remain up-to-date across portfolios
- Trade execution monitoring, refining best execution strategies with real-time insights
Hedge funds need a high-speed, unified platform that can process massive datasets efficiently while maintaining low-latency performance.
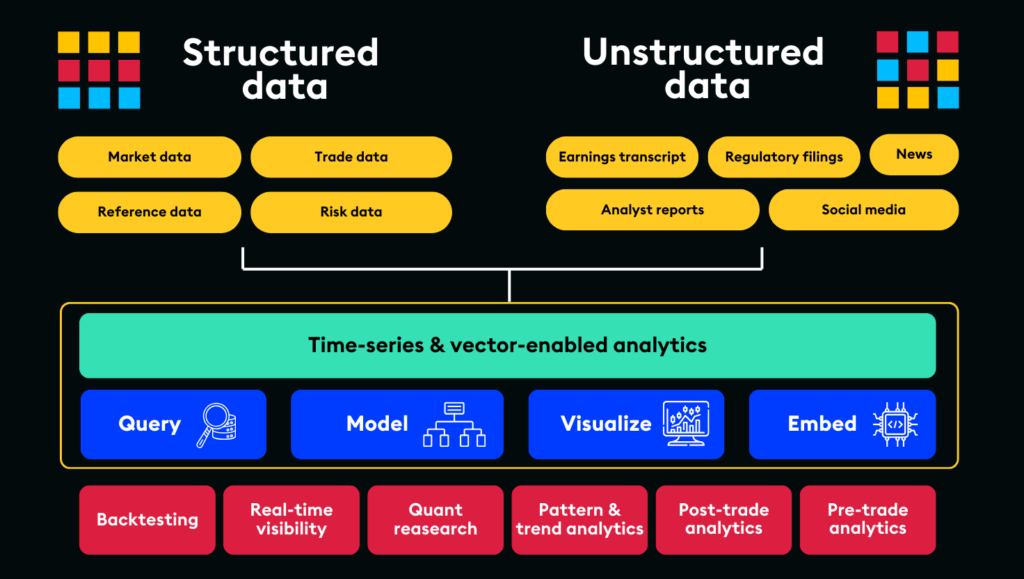
Delivering timely insights for hedge fund analytics
While speed and low latency remain important in hedge fund analytics, they are not the ultimate drivers of alpha. The ability to generate high-quality, timely insights — delivered with precision and context — is what separates top-performing hedge funds from the rest.
This is where kdb Insights provides a powerful advantage. Designed for pre- and post-trade analytics, quant research, backtesting, and pattern and trend analytics, kdb Insights enables hedge funds to:
- Process real-time and historical data seamlessly, ensuring that market signals are validated before execution
- Optimize trade execution analysis, identifying slippage, liquidity risks, and post-trade inefficiencies
- Enhance backtesting workflows, eliminating look-ahead bias while maintaining high-speed performance
- Enable AI-driven trend detection, refining factor models and strategy adjustments in real time
- Deliver high-performance time series analytics, supporting rapid decision-making without compromising accuracy
Explore how KX empowers hedge funds with context-rich analytics, seamless data integration, and AI-ready infrastructure.
Discover how leading hedge funds stay ahead with real-time analytics — download our ebook for in-depth insights or complete our hedge funds analytics checklist to uncover key opportunities to enhance your trading edge.