In this blog, Mark Palmer introduces us to the ‘AI factory’ and explains how it systematizes the culture, processes, and tools required to apply AI solutions within an organization.
This is the first part of a series of “AI factory 101” posts where Mark Palmer describes the elements of an AI Factory and how any organization can implement one to catapult your organization into a fast, agile, innovative, healthy factory of AI algorithms. Read part two here.
Netflix transformed the media industry by harnessing the power of AI.
At its core is an AI-centric operating model powered by software, organizational constructs, and cultural design. It facilitates data collection, algorithmic discovery, and AI deployment that influences virtually every aspect of the business, from personalizing the user experience to picking movie concepts to negotiating content agreements. As Jory Evers said, “There are 33 million different versions of Netflix.”
The secret of Netflix’s success is how they use technology to create such an adaptive, personalized, automated experience. This is the future of business in the AI age. But do you have to be Netflix to harness AI in this way? The answer is no, but you do need to rethink organizational design, culture, decision-making approach, and the tooling required to support them in the AI age. In Competing in the Age of AI, authors Marco Lansiti and Karim Lakhani call this modernized approach to business the “AI Factory.”
In part one of this multi-part series about the AI Factory, we’ll explore everything you need to know about how to implement your own AI-age innovation system and why they matter.
The secret of effective AI culture
The path to AI innovation is paved with processes, tools, and culture that help answer questions quickly, experiment with algorithms, and codify ones that work continuously, adaptively, and securely.
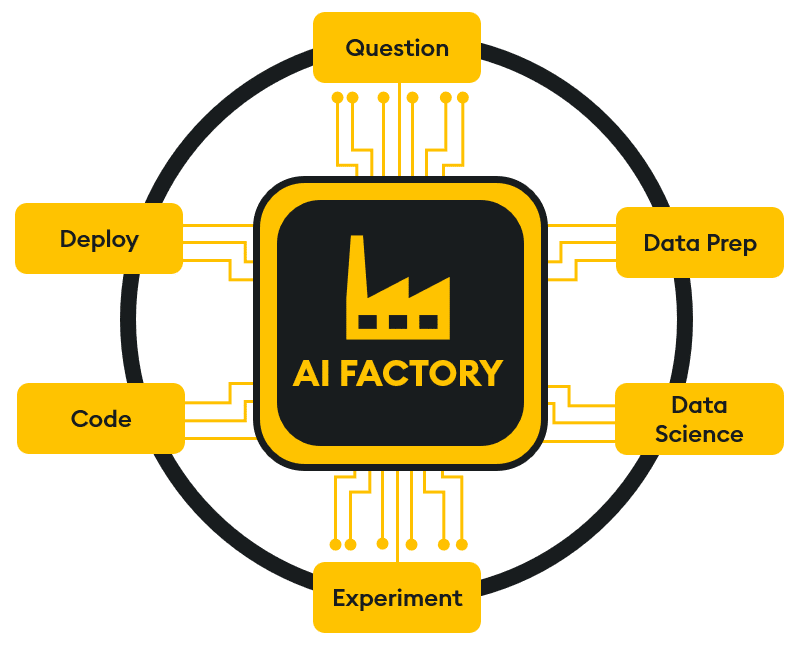
Dana-Farber Cancer Institute tested this idea. They used AI to characterize lung cancer with two “citizen data scientists” – physicists with no medical imaging background – to create a transformative application that diagnoses cancer faster than a human-only approach.
They set a clear “North Star” objective, organizing training data from 461 patients and over 77,000 CT image slices. The team created an experimentation playground for participants (none of whom had prior experience with cancer diagnosis), built a prototype system, and deployed the most promising AI algorithms.
The resulting system mapped the shape of lung cancers at rates between fifteen seconds and two minutes per scan – substantially faster than a human expert, who took eight minutes per scan. The ensemble of the five best algorithms performed as well as a human radiation oncologist and was better than existing commercial software. The results were so compelling that the team published their findings in The Journal of the American Medical Association Oncology.
Most organizations aspire to be this AI-agile. Yet, according to Wavestone’s 2024 Data and AI Leadership Executive Survey, only 5% of senior leaders report deploying AI at scale in this way. So, we have some work to do!
Most organizations don’t have the luxury of designing a clean-sheet environment to apply AI to business problems. Instead, large, siloed, complex, bureaucratic, misaligned, arcane, outdated IT systems reign. But the Dana-Farber experiment illustrates the art of the possible and paints a vision of what an AI factory can accomplish.
An AI factory helps scale an algorithmically aware culture
Scale comes from processes, culture, and tools that support business, technical, and analytics teams, the right collaboration around the change it imputes, and encouraging the spirit of curiosity, experimentation, failure, and learning to unlock new possibilities with artificial intelligence.
Here’s how it works.
Part one: AI factory 101
An AI Factory systematizes the culture, processes, and tools required to apply AI. But while the metaphor of a factory is useful, we’re going to mix two metaphors explicitly.
That is, the factory we want is more like a commercial kitchen because we’re not trying to produce any old widget or inanimate object. No, the “factory” we want is like a restaurant: our goal is to produce inspired, creative tastes, aromas, and an innovative dining experience. This experience, like a memorable meal, makes for an elevated business experience that surprises, delights, and delivers on customer expectations.
Our AI factory – our kitchen – has five stages that produce these unique business experiences. The stages include:
- Data Pipeline: The AI Factory starts with data like a great restaurant starts with the best, freshest, highest quality spices, fruits, and vegetables. Similarly, an AI Factory begins with clean, quality, prepared data.Data pipelines ensure ingredients are processed carefully for consistent quality. Data pipelines are the first stage of the AI Factory journey. It ensures we’re ready when it’s time to get cooking.
- Algorithm Development: Once our data is prepared, we cook. Data scientists are our chefs; data engineers are our sous-chefs; business leaders are the executive chef. In a kitchen, executive chefs set the menu; leaders set OKRs and business objectives in an enterprise. In a kitchen, the chef uses instinct, speed, and skill to turn ingredients into the perfect dish; in the enterprise, data scientists do the same with data.
- Experimentation and Experience: Experimentation is the most overlooked secret to business and culinary success. Hours, weeks, or even years of experimentation happen before we perfect our food and dining experience. In the enterprise, an AI Factory is designed with experimentation in mind: to taste recipes early, often, and continuously. To add salt. To declare failure and start all over again at any time.
- Software Infrastructure: Finally, we consider the tools of our trade. Like the design of a dining room, staff selection, and implements like knives, appliances, plates, and glasses, the tools we use in an AI Factory define our business. Unlike a restaurant, the AI tools used by large enterprises are more capable and primary to an AI Factory than a knife. But, treating them as tools and supporting our overall endeavor is the right way to think about the software infrastructure required to produce an effective AI factory.
- Deploy and learn: Finally, we deploy, test, learn, and adjust. Like a restaurant, a business is ever-changing, ever-adapting, and forever sensitive to the reaction of our clientele. We try, listen, and adapt our recipes and the context in which we deliver them. Our AI Factory must support this process of being agile and adjusting our approach based on the feedback about our results.
Ultimately, our AI Factory kitchen looks like this with data entering our system, algorithms developed, and AI-driven insights, like a great, creative decision-making meal served to patrons quickly. Supporting it all is our software infrastructure.
How to Build an AI Factory
You don’t have to be Netflix to build an AI factory. But the Dana-Farber experiment paints a vision of what mainstream companies can achieve when data pipelines are clean, and teams have a focus and a bias for action.
This article is the first in a series of five blogs that describe the elements of an AI Factory and how any organization can implement one to catapult your organization into fast, agile, innovative, healthy factory of AI algorithms. Read part two here.